There is no “super algorithm” that will work perfectly for all datasets.
Models are simplifications of a specific component of reality (observed with data). To simplify reality, a machine learning algorithm or statistical model needs to make assumptions and introduce bias (known specifically as inductive or learning bias). This phenomenon is important to understanding the concepts of underfitting and the bias/variance tradeoff.
The combination of your data and a randomly selected machine learning model are not enough to make accurate or meaningful predictions about the future or unknown outcomes.
You, the human, will need to make assumptions about the nature of your data and the world we live in. Playing an active role in making assumptions will only strengthen your models and make them more useful, even if they are wrong.
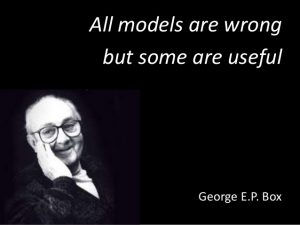
Comments